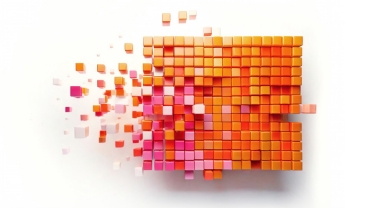
12 May, 2023
Artificial Intelligence (AI) has come a long way over the last few years, breaking out of the labs and universities and establishing itself in almost all industries and in the conscience of the general public. Somewhat lesser known than its advantages through superhuman abilities and irrational fears fueled by science fiction stories about AI robots taking over the world are realistic and unintuitive security threats, also known as adversarial attacks.
These threats prey on the key strength of AI systems – their autonomy. In order to utilize the benefits of AI without having to worry about the risks associated with adversarial attacks such as Data Poisoning, Model Theft, Evasion- and Inversion Attacks, organizations must patch their risk management processes to make them fit for AI threats. Learn more about how to do that by contacting our specialists working on AI security and Robustness.
Due to its reliance on information in huge datasets, AI is an immensely powerful technology, capable of solving tasks that conventional IT systems are not able to tackle. The freedom granted by developers to flexibly find out how to solve the task at hand by itself however also leads to vulnerabilities, since the behavior of AI systems, especially when operating on high-dimensional data like images, text, or sound, cannot practically be predicted for all possible inputs. Hence, trustworthiness is essential for business adoption of AI: developers must demonstrate that, even though one cannot guarantee the intended functionality of the AI system for every conceivable input, the risk posed by such malfunctions is reduced to an acceptable level, which of course depends on the specific use case. In the future, such requirements will increase due to the evolution of AI regulation.
Data Poisoning
Data Poisoning describes an attack on the functionality of AI systems, where an attacker manipulates the data set used for training of the AI model before the latter takes part, or in the case of continuously learning models, during operation (or a combination of both). In general, the magnitude of the effect of data poisoning attacks is directly proportional to the fraction of poisonous data injected into the data set used for training.
Model theft
Model theft describes the attempt to extract the model parameters, and thus the model itself, by engineering certain sets of inputs, imposing these on the model, tracking the corresponding outputs to obtain labels, and in combination with some preliminary knowledge of the model architecture, reverse-engineering the AI model. Model theft can often be achieved with surprisingly little effort, where in some scenarios researchers found that models can be extracted with fewer than 1/5th of the queries that have been used to train the model in the first place.
Evasion attacks
Evasion attacks target AI models with malicious, deliberately constructed inputs, which are known as adversarial examples and lead the model to exhibit unintended behavior. Evasion attacks can be performed in white-box or black-box contexts, indicating the amount of information an attacker can access. While black-box attacks still have a very low success rate (about 4%), a well-planned white-box attack (e.g., following the fast gradient sign method) is almost always successful.
Inversion attacks
Inversion attacks attempt to reconstruct data that has been used for training a given target AI model. Preventing model inversion attacks is especially crucial for AI system providers dealing with sensitive user information, since substantial reputational damage is to be expected in case of failure to prevent this threat.
To find out how to defend your system against these attacks, download our whitepaper on AI security and robustness.