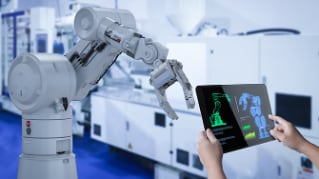
Digital Twins will change the way you look at your business
Digital Twins provide organizations with a new era of decision making and planning.
Businesses are always seeking for an elevated level of intelligence and control over their operations, leading to greater efficiency and productivity. To remain competitive in today's rapidly evolving business world, it is essential to keep up with the latest technological advancements. The emergence of AI technologies, including foundational models like ChatGPT, has demonstrated their potential to revolutionize the way we work. Digital Twins and Reinforcement Learning are two highly impactful technological advancements that have emerged in recent times with the same revolutionary potential.
By leveraging technologies such as Digital Twins and Reinforcement Learning businesses are able to gain competitive advantage by lowering costs and improving quality and safety, e.g. of production processes.
In contrast, by not embracing these technological changes and keeping up with the pace of innovation companies may risk falling behind competition. The possibilities for leveraging such technologies seem limitless, so it is time to elevate your business to the next level.
Digital Twins virtually replicate real-world objects and enable you to visualize your data nicely, e.g. in a metaverse. They can be set in reference to each other and interactions between these are framed as Simulation. Imagine, for example, the production of a screw. Sounds like an easy process but many parameters must be managed in order to get to the right production quality. The machine can be represented by a Digital Twin as can be the metal blank. When the machine transforms the blank into a screw the two components interact, as do their Digital Twins to control the process. In other words the real-world process is simulated virtually.
“AI has emerged from the scientific castles and is ready to conquer businesses and society all over the world. Now is the time to adapt and succeed in this exciting journey.”
A wide range of Simulation tools modeling these Simulations explicitly already exist. Despite this, building these Simulations can often be difficult, tedious and time-consuming due to the limited explicit knowledge about the possible interactions between the components and hence the Digital Twins. For example, not all processes and interactions that influence the transformation of the blank are known, let alone can be explicitly modeled, e.g. due to effects of environment pressure or blank quality. This shortcoming can be overcome using AI.
Building on the data foundation of the corresponding Digital Twins the complex influencing factors do not need to be explicitly modeled, but are learned by the AI. This creates AI based Simulations requiring only the acquisition of data and saves time, e.g., the time-consuming Simulation modeling. Thus, AI technologies based on Digital Twins represent a turning point for companies that want to increase their efficiency and productivity and fully exploit the potential of their digital transformation.
Also, an AI-based Simulation is a suitable foundation for Reinforcement Learning (RL). This type of AI learns from past experience by interacting with its environment, rather than relying solely on labeled sets of data. This makes it well-suited for tasks where the optimal actions, e.g. machine parameters, are not well-defined or difficult to determine a priori. Recalling the screw example, an AI trained in the simulated environment can then be deployed on the real-world machine autonomously producing optimal screws without expensive human intervention. The combination of Digital Twins and RL thus has the potential to revolutionize the way business decisions are done. To back this up, surveys show that 62% of companies using RL already benefit after a maximum of three months.
Before diving into the benefits of Reinforcement Learning let’s have a rough look into the engine room: Reinforcement Learning is a subfield of AI and (in its original form) separated from the well known machine learning paradigm (see below).
The RL set-up consists of an environment in which a so-called agent executes actions. Recalling the example from earlier, the screw production machine could be controlled not by a human being but an AI entity, the agent, that adapts the machine parameters (actions) such that always the desired quality is produced. In a static environment without variances, e.g. in the blank quality, machine or environment temperature, this replacement does not provide much business value. In general though these assumptions do not apply and the screw manufacturing machine is embedded in a real world environment with changing conditions. Like a human being, the agent has to learn how to adjust the machine's parameters to different environmental conditions for consistent quality. The agent learns choosing the optimal machine parameters through interaction with the environment during training and thus from experience.
The great advantage of an RL agent over human-based machine parameter adjustment for every single screw is a production without delays, e.g. for setting up the machine. Also, the agent works all day round at the same speed and quality, increasing productivity and leading to less human errors. For a technological deep-dive please be referred to our personal favorite below – the “AI-Enthusiast section”.
To further improve the advantages of RL it can be combined with the machine learning paradigm. One popular approach is Deep Reinforcement Learning (DRL), which integrates deep learning as part of the machine learning paradigm and Reinforcement Learning. In DRL, the agent is represented by a neural network that learns how to control the machine. The major advantage is the ability to detect hidden patterns for controlling the machine that are too complex for a human operator to comprehend.
The ability to autonomously find solutions and hidden strategies for complex tasks that would otherwise require intensive human intervention makes DRL a distinguished technology in the AI field. This type of automation leads to greater efficiency, higher and steady product quality and better robustness against process variations (like in the screw production example above).
These benefits can also be utilized, for example, to reduce the lack of well-trained staff. Besides finding well trained staff, e.g. for production assembly or welding, being an expensive and time-consuming task, RL can also reduce the dependency on these highly specifically skilled personnel. Another great advantage of RL is that significantly less data is necessary for creating an agent-controlled welding robot compared to classic machine learning approaches, e.g. computer vision for facial recognition. The reason is RL’s ability to develop its own solution strategy rather than being presented with the solution to be learned, e.g. what does a face look like. For sure the screw manufacturing machine represents only the tip of the iceberg, as there are infinitely more applications of DRL across all industries in development and already in use.
In a smart manufacturing facility, a Reinforcement Learning system can be employed to optimize the production process of electronic devices. The RL system interacts with the machines, adjusting parameters like machine speed, temperature, and material usage to maximize production output while minimizing defects and resource consumption. Through continuous trial and error, the RL system learns and refines its strategies, adapting to changing conditions such as raw material variations or market demand. As a result, the smart manufacturing system achieves higher production efficiency, reduced defects, and improved resource utilization, leading to enhanced profitability and competitive advantage.
All these applications are proof of RL's potential, technological readiness and its ability to create real business value across a wide range of industries. In addition, and among the most important, a good understanding of RL from an academic and business perspective has made the risk in developing RL systems highly manageable.
The advantages of reduced costs and time by using RL can be increased even further by optimizing the training phase of RL models. Training RL models in a real-world environment is often challenging due to high costs, lots of time needed and risks involved. For example, using a real welding robot for training works, but causes high material costs and most likely damage to the robot itself and takes a lot of time. However, the RL concept can also be utilized in virtual environments that simulate real-world conditions well enough. This enables agent training without the risk of causing serious damage and again massively decreases costs. The virtualization of training further enables training of multiple agents in parallel decreasing training times and thus, also, costs even more. For example, training an agent for autonomous driving virtually avoids potentially costly and dangerous risks in the real-world. After training in the virtual environment the agent is deployed in real-world acting according to its learned policy. The cascading development steps embedded in agile project management shorten the time to ROI and minimize process risks, as adjustments can be made quickly and regularly in project implementation.
“AI has gotten to the point where it is ready to be applied in real-world products. Using it, things are possible that seemed impossible not long ago. It’s great times offering great opportunities.”
The agent's applicability in the real-world mainly depends on how well the virtual environment replicates the real-world, what is known as simulation-reality-gap. In general, it is not necessary and also very costly, to create a virtual environment that replicates reality in an exact manner. Instead, it is more important to develop an environment that creates a sufficiently complete picture of reality while omitting unnecessary information. Such a focus on the essentials requires a good understanding of the real-world. In the end, it leads to an appropriate balance between accuracy and cost-effectiveness in order to make the best use of the advantages of the virtual environment.
A great methodology to get to the sweet spot between cost and accuracy are Digital Twins. In the context of RL Digital Twins represent the real-world entities and serve as the virtual environment to train the agent. Additionally, the agent itself can be represented by a Digital Twin of a real-world entity, e.g. the screw manufacturing machine. This digital representation of the real-world through real-time updated Digital Twins enables well trained agents that are highly performant during their deployment in the real-world. The semantic data structuring and real-time update through Digital Twins allows DRL algorithms to be trained in a safe and trustworthy virtual environment. This foundation then enables the development of DRL models.
Digital Twins provide organizations with a new era of decision making and planning.
The challenges of applying AI in medical devices and how they can be addressed along the AI lifecycle.
Discover how Digital Twins and Reinforcement Learning drive innovation and transform industries. Stay ahead with the latest technology advancements.
For AI transformation, we need clear competitive conditions and responsible, trustworthy solutions. PwC supports you in this.