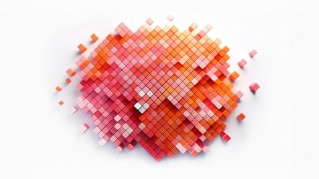
Preparing your Data for GenAI
The experts at PwC elucidate why data is essential for the successful deployment of GenAI and guide you on how to optimally prepare your data for this purpose.
Written by Hendrik Reese, Jan-Niklas Nieland and Daan Herpers. Artificial Intelligence (AI) is not just a form of technological innovation; it is a profound redevelopment of how humanity interacts with machines and data. With its increasing reach and impact on society, AI development carries a strong ethical imperative that transcends specific sectors. In this rapidly changing field, where use cases seem boundless, we find ourselves at a critical juncture in human history.
AI´s transformative power demands us to reflect on many ethical questions: How do we ensure fairness and trustworthiness in AI systems? How do we mitigate bias and discrimination? How do we protect individual privacy when interacting with data intensive operations? How do we safeguard against misuse and malevolent intent?
As we delve into the topic of responsible AI development and usage, one must acknowledge the specific opportunities and challenges the domain of generative AI (GenAI) has brought forward. GenAI is the newest wave of development in AI and digitization in a broader sense, having the capability to analyze and learn the patterns of large data sets and apply those to generate new content following a similar structure. Large Language Models (LLMs) exemplify this by generating coherent and contextually relevant language. GenAI features great improvements over other forms of AI when it comes to input and output flexibility. This has led to the rise of many popular tools made for a consumer market. Where it took Netflix 3.5 years to get 1 million users, ChatGPT did it in only 5 days.
Because GenAI possesses the ability to create diverse outputs across sectors and countries, we see entire economies being impacted. Consequently, these advancements raise a host of ethical challenges that must be grappled with to ensure they are harnessed for the betterment of society. These include, but are not limited to dilemmas regarding intellectual property, issues of bias and fairness in generated content, the spread of misinformation and manipulated content, and accountability and transparency issues. Finding ways to operationalize trustworthy solutions to these multifaceted issues is therefore not only a moral imperative but a necessity for ensuring that generative AI fulfills its global transformative potential for the benefit of societies.
GenAI’s generalizable functionality and resulting large consumer base amplifies the aforementioned ethical challenges and has led to a greater call for transparency and accountability to increase the explainability of generated outputs so errors, biases, or malicious use can be identified more confidently. Transparent GenAI systems enable all stakeholders to find out how its content is generated and comprehend its limitations, which fosters a trustworthy relationship between GenAI and society.
The importance of transparency and accountability has been reflected in (developing) regulatory frameworks. The EU AI Act establishes transparency requirements depending on the risk the system has on society, for GenAI this means stronger transparency obligations, publicly available information on the training data and the registration of the model in an EU database. The work on standardization both horizontal and sector specific for AI systems is equally as important. Reliable frameworks give industry partners the orientation they need for establishing internal roles and processes for accountability and knowing what information has to be shared with stakeholders. Before many of the responsibility issues of GenAI can be addressed, we need to establish a (governance) ecosystem in which transparency is nurtured and accountabilities are assigned.
At the front end of many GenAI tools, it may seem like AI is getting smarter and more intelligent. Even though it seems like these tools can sometimes outperform human intelligence, they still need data from us as a reference to display, what we call, intelligent behavior. These models learn from large datasets, which often mirror the unconscious biases (e.g. related to race, gender or age) embedded in human behavior and society. AI models fully relying on historical data perpetuate those biases within the output they generate, which can reinforce harmful stereotypes and discrimination. An example is hiring algorithms that favor male candidates for technical positions, as they learn that those positions are often filled by men by humans from the data it is fed. Such a system uses biases in our world and makes the gender of the applicant a decisive factor, but this is not always being explained to the users of the system. This is why transparency and explainability is important, diverse, representative datasets should be used, and biased data points should be identified and corrected. Techniques like bias elimination and adversarial training can be employed to mitigate bias and improve fairness in the outputs.
Fairness considerations in model outputs or decisions are essential for ensuring unbiased outcomes. When a model makes a decision, it should not favor one category of sensitive personal data over another, e.g. an applicant's gender when rating job applications. This seems logical, however, achieving this is easier said than done. The biases leading to the unfair treatment of individuals are not visible to the system’s users as it is unclear which factors resulted in the AI system’s output. Additionally, fairness is not a numerical value easy to measure but a mere interpretation of the output at hand. Because of this, general ethical standards acting as guidelines for developing and evaluating the fairness of an AI model are needed, which can come in the form of Code of Conducts. Apart from that model outputs should be carefully monitored and tested for disparities among different relevant groups. Machine learning fairness metrics, such as demographic parity, equality of opportunity, and equalized odds, can be employed to assess and improve fairness. Additionally, standards for bias correction should be established in model training. As these issues also touch on the human side of development, it is important to establish dialogue with domain experts and affected communities to identify and rectify potential fairness issues.
Addressing bias and fairness plays a central role in GenAI, as they are often intransparent in nature and function as a blackbox. While the input (data) and the output (generated content) are visible, the internal processes are complex, opaque and mostly hidden. This is a significant hurdle for trust, accountability and addressing biases. Because of this, explainability measures to establish the capability of describing how a certain decision was reached are essential for GenAI. To enhance explainability, model architectures need to be interpretable by relevant stakeholders. So called ‘explainability algorithms’ can be implemented and transparency features need to be adopted. Opening the blackbox of GenAI by using adequate tools should also involve designing intuitive interfaces to establish meaningful explainability for its diverse user base, including non-experts.
For answering to the issue of bias and fairness issues in GenAI, robust models need to be developed. Outputs should be monitored, and mechanisms for feedback and reporting have to be in place to prevent the amplification of harmful or biased content. As not all AI models pose the same risk potential, use cases need to be assessed individually, for instance through the risk-based classification approach of the European AI Act. AI systems that can have a significant impact on people or are applied in critical infrastructures have to comply with stricter requirements. Apart from that, the European Commission also started a stakeholder survey to define guiding principles in the form of a voluntary G7 Code of Conduct for the trustworthy development of AI tools on a global level. Besides binding AI-specific laws, frameworks like the BSI’s AIC4 in Germany, the AI Risk Management Framework by the National Institute of Standards and Technology (NIST) in the US or the Microsoft Responsible AI Standard try to set measurable standards for the responsible development and use of AI systems.
To ensure the responsible use of GenAI within organizations, concrete specifications and company-wide guidelines become paramount. Such guidelines can offer support in defining permissible application areas and purposes (for example, for generating or summarizing text, for translation, in customer service, or for revising code) and identifying the risk potentials of such systems. Guidelines should establish clear accountabilities and transparency requirements. Another important factor that needs to be taken into account is the trust of internal as well as external stakeholders in a company’s application of GenAI as well as the regulatory landscape that is relevant to the specific AI use case.
To ensure a responsible handling of GenAI within the staff, it is essential to educate employees about the opportunities as well as the risks that can arise when applying GenAI, such as reputation loss, legal issues, or security concerns. Employees should also receive training on quality improvement opportunities, e.g. prompt engineering, to enable productive utilization along with training on system vulnerabilities, so employees understand the need for independent verification and the dangers of publishing sensitive information. Where possible, employees should be involved in reflecting on and designing the used GenAI tools, as they can have necessary knowledge on how such tools are and should be involved.
In external communications, a Code of Conduct that is accessible to customers and other stakeholders is useful for increasing trust. In this Code of Conduct, organizations can offer information and explain the areas in which GenAI is and is not used. Additionally, it is advisable to disclose which content or other output was created with the help of a GenAI model and when customers are actively interacting with a system, for instance in the form of a chatbot. Feedback from external stakeholders can be a necessity for optimizing applications and offering user-centered services.
Regulatory bodies play a fundamental role in upholding responsible AI practices. Apart from these organizational efforts to assure the responsible use of GenAI, regulation should support the enforcement of societal values, foster innovation and offer best practices. The reoccurring AI safety summit, shows such efforts to establish international collaboration for regulating frontier applications such as GenAI. The boundlessness of GenAI can only be meaningfully addressed through such collaborations.
Despite this, laws and regulations alone will likely not suffice in fighting against the potential risks of GenAI, especially with regard to cyber attacks, hackers or fake content. Therefore, organizations cannot solely rely on upcoming regulation like the EU AI Act or an AI bill of rights from the US, but need to put their own security measures and standards for responsible AI use into place. Standards for trustworthy GenAI applications can offer companies competitive advantages, establishing certification possibilities and therefore enhancing public trust in GenAI solutions. Collaboration between public and private parties remains pivotal in shaping a future in which trustworthy practices are embedded in the very fabric of AI innovation.
Our role as PwC is to help you navigate through this complex web of business, regulation and technology by unifying our industry expertise with valuable insights and experience to create a holistic picture and actionable best practices to drive your AI transformation in a trustworthy and responsible way. Our proven AI Governance framework encompasses all relevant stages from development to operation and responsible use. We make use of our industry-wide network to not only help you establish state-of-the-art use cases in your organization but also apply them in a trustworthy way.
Christine Flath
Leitungsteam Familienunternehmen und Mittelstand und Ihre Ansprechpartnerin für Transformationsthemen, PwC Germany